Empowering Alternate Data Collection for Digital Lending with Account Aggregator Framework - Challenges & Solutions: PART - 2
In Part 1 of our blog, we talked about the importance of alternate data and the role it plays in making lending easier for the large segment of the untapped population thereby leading to financial inclusion. Now, let’s look at the possible hurdles in achieving our goals of financial inclusion.
Challenges in gathering and using Alternate Data
While alternate data is a step in the right direction, it’s still not easy for many organizations to be able to extract it and put it to good use.
- Privacy, Transparency, Control -> Trust:
- There are a lot of mobile apps from fintech companies that upload the mobile data (SMS, contact list, call logs, location) gathered from their customers to their servers frequently. This data is used by the organizations to calculate the propensity to pay, offer them new credit products based on their eligibility and establish a contact in case of delinquency.
While customers do give the required app permissions when they first install the app, the specific details of what data is being used is either written somewhere in the small fonts or completely missing altogether.
A few mobile apps even Additionally, data privacy was also highlighted as a big challenge in the World Bank’s Global Symposium on Microfinance (2017).
One lender sharing the late repayment data of a customer with others in the ecosystem, without taking into account the circumstances that lead to the delays in the first place, can lead to denial of credit from all the banks. - While the customers who are in genuine need of credit would find it acceptable to provide access to their mobile or social data, neither they have much control over what specific data they can share nor the duration for which they want to share the data. In the same context of mobile apps of fintech organizations, one of the resorts is to uninstall the app altogether. But by doing so, they are also deprived of other features of the app (like repayment reminders).
- There are a lot of mobile apps from fintech companies that upload the mobile data (SMS, contact list, call logs, location) gathered from their customers to their servers frequently. This data is used by the organizations to calculate the propensity to pay, offer them new credit products based on their eligibility and establish a contact in case of delinquency.
- Quality and Fragmentation of Alternate Data:
- For the organizations looking forward to utilizing alternate data, the biggest impediment is the format in which the data is available. A simple problem statement of reading the bank credit/debit information from the PDF statements is a very hard one to solve given the fact that each bank has a different bank statement format and a lot of banks also password protect the statements.
While companies like Perfios do have bank statement parsing solutions available, it requires customers to go through multiple steps to upload a simple statement thus eventually leading to customers dropping off. To solve this, financial institutions have to introduce additional operational steps which leads to a break in a digital journey and thus to an increased TAT. - Parsing SMS data so that it can be used as a bank passbook is another hard-to-solve problem that requires a good investment in AI/ML-based models and multiple iterations to get it right.
- For the organizations looking forward to utilizing alternate data, the biggest impediment is the format in which the data is available. A simple problem statement of reading the bank credit/debit information from the PDF statements is a very hard one to solve given the fact that each bank has a different bank statement format and a lot of banks also password protect the statements.
- Overarching bias and the risk:
Given that there is a heavy reliance on technology for using the different variables in alternate data and building AI/ML-based models on top of it, there are not enough regulations in place to prevent any bias which can inadvertently be induced by using variables like race, sex, religion, ethnicity etc.
Additionally, there is a risk that some of these sophisticated tools may be used to target more vulnerable customers towards predatory lending. These are the important concerns raised by Hurley & Abadayo in their Yale Journal of Law and Technology (2017).
AA Framework: India’s first step towards Open Data
One of the policy recommendations by the Global Partnership of Financial Inclusion (GPFI) in the G20 summit in 2018 was :
“POLICY RECOMMENDATION 5: OPEN DATA SYSTEMS AND STANDARDS
Policymakers should explore the option of developing an Open Data System and Data Standards for MSME data (including corporate, financial, banking and other relevant alternative data).”
PSD2 in the EU is a great example of opening up the banking and payments data by sharing the same across banks and third-party providers while passing the control in the hands of the customers. Back home AA Framework is a first step towards doing the same. Even though currently customers can start sharing their Assets data with third parties, the vision is to move towards an open data economy and empowering Indians with control of their financial data.
While the AA framework may not be able to solve all the challenges listed above, it’s a step in the right direction to solve
(1) Privacy, Transparency & Control
(2) Quality and Fragmentation of Alternate Data hurdles.
Lending App Use Cases with AA Framework
Now let’s look at a use case with AA Framework in action in order to have the customer’s perspective. But before moving ahead, here are a few assumptions to make:
- CleanLend is a (fictional) digital lending fintech (FIU) that provides short term personal loans to customers for the loan amount in the range of Rs 10,000 to Rs 2,00,000 and tenures ranging from 6 months to 2 years. Customers have to download the CleanLend app from the Google play store and go through the app journey of sharing their details and upload documents before the loan could be disbursed to their loan account.
- The target customer base of CleanLend is the customer in the monthly income bracket of Rs 10,000 to Rs 50,000.
- CleanLend has been using a 3rd party bank statement analyzer to find the income of the customers when they upload the PDF statement of their bank statement. But they have observed 50% customers drop-off; the biggest contributors to the drop-off have been :
- Customers have an image of passbook but not the PDF format
- the customer is not able to generate an e-statement
- the statement provided by the customer is not in the format as supported by 3rd party bank statement analyzer.
- CleanLend has implemented AA’s FIU module and its lending use case is using the AA rails in the CleanLend mobile app.
User Flow on CleanLend’s Mobile App; powered by Account Aggregator Framework
1. Customer lands on Create Account screen of CleanLend app
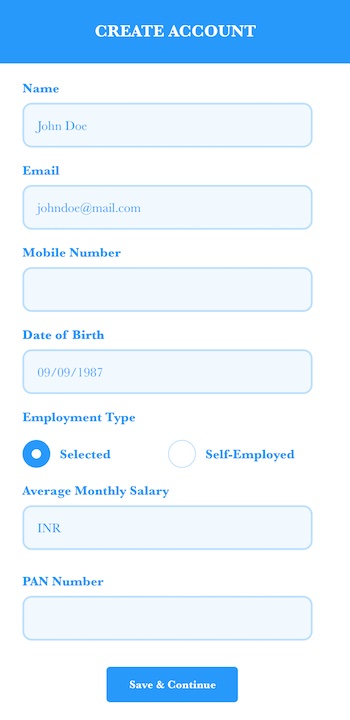
2. The customer’s mobile number is verified by an OTP
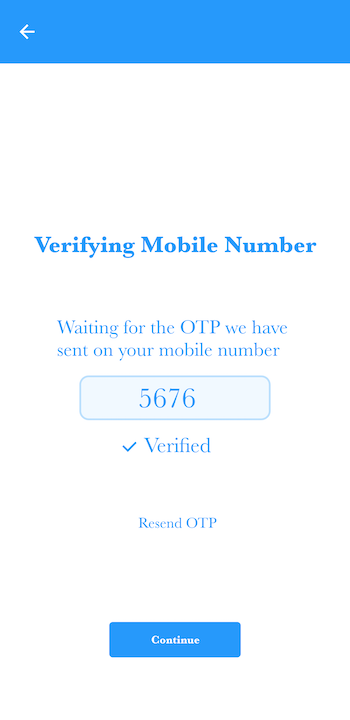
3. Customer’s bureau data is checked using CIBIL
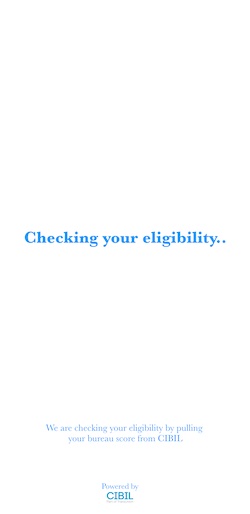
4. Since the customer is NTC (new to credit), there is no hit against the bureau and as a result, the customer is asked if they would want to share the bank details instead.
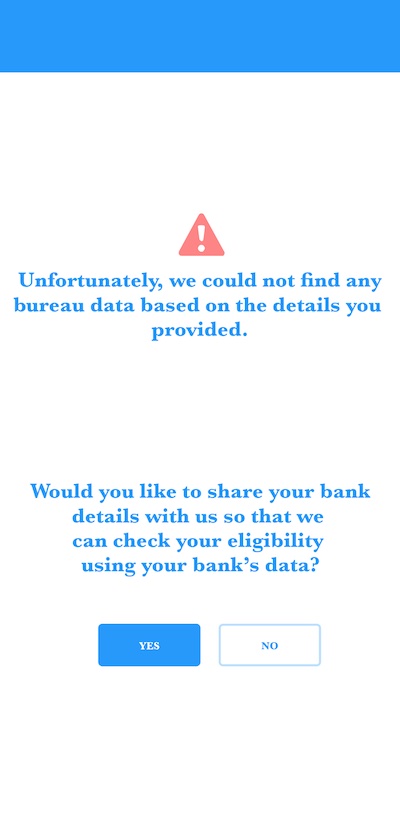
5. The customer is shown the available list of banks
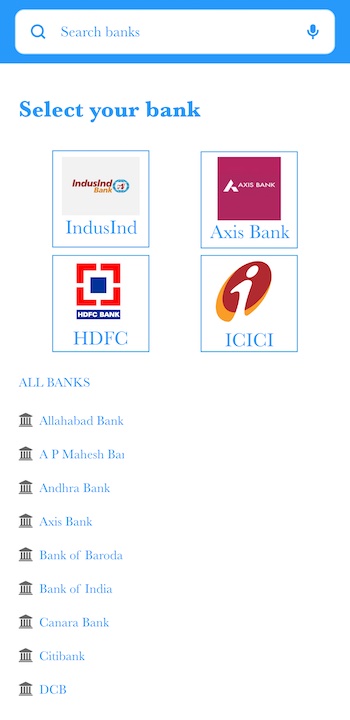
6. The customer chooses a bank where they have an account(s)
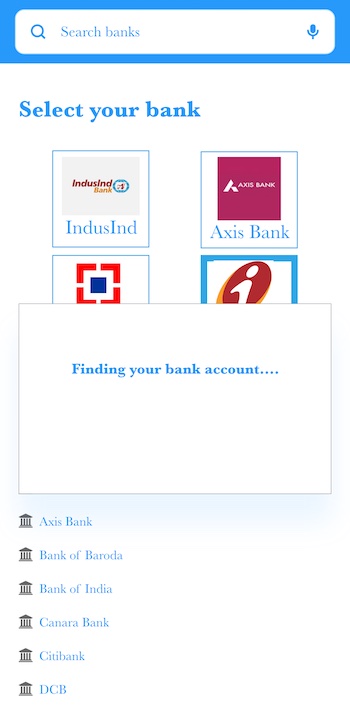
7. The bank account(s) linked with the mobile number is shown to the customer
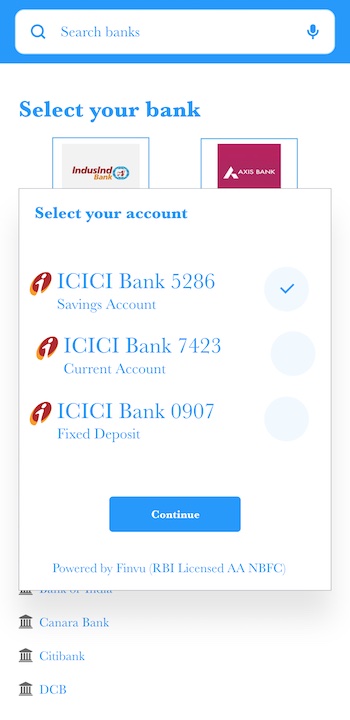
8. The customer’s loan eligibility is checked.
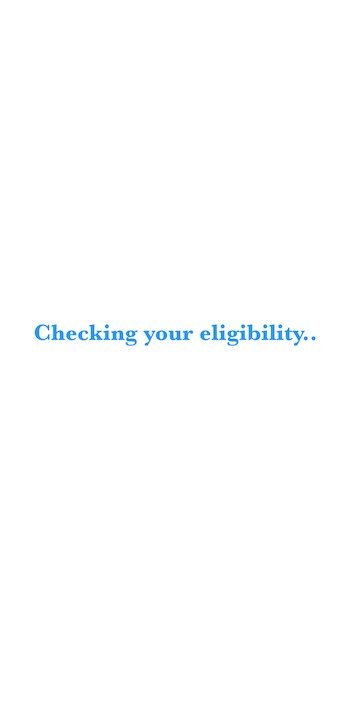
9. Based on the customer’s bank account details fetched, the customer’s loan is approved. The customer is also shown an option to provide access to any other bank accounts if they wish to have a higher loan amount.
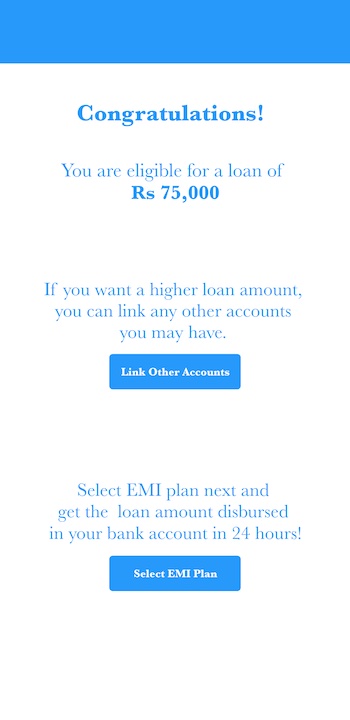
10. The customer chooses to give access to other bank accounts:
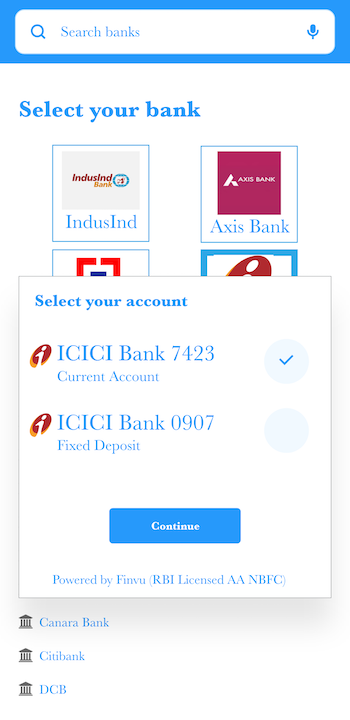
11. The customer’s eligibility is checked again and is now provided approval of a higher loan amount based on the newer information shared.
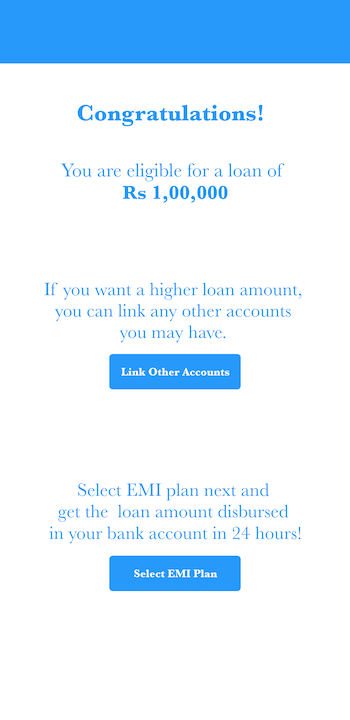
Conclusion
The use case here shows that a customer who may have otherwise been turned down because of non-availability of credit history, or in other words, having no bureau score, is now taken down the path of sharing their bank’s details thereby serving their lending needs.
It’s important to note however, that the above mentioned use cases, tries to solve the financial exclusion problem by having a more streamlined way of gathering alternate data for customers who are considered New To Credit (NTC) or in lending parlance, have thin files. In our future posts, we shall also cover how AA further strengthens the underwriting capabilities of the lenders for customers who are visible to the credit bureaus.
References:
- Universal Financial Access 2020, The World Bank
- Financial Inclusion Overview, The World Bank
- Need for Financial Inclusion and challenges ahead – an Indian Perspective, iosrjournals.org
- Private credit bureau coverage (% of adults) – India | Data (worldbank.org)
- Report of the Committee on Financial Inclusion, January 2008, slbckarnataka.com
- How to Measure Financial Inclusion (worldbank.org)
- Alternative Data and the Unbanked (oliverwyman.com)
- Dec 2020 Insight Report_R4 copy (transunioncibil.com)
- Lenddo-FS-CreditScore-v4
- Experian Boost – Improve Your Credit Scores Instantly for Free
- India’s Financial Firms to Increase Investment in Alternative Data Sources, TransUnion CIBIL
- Revolutionizing Microfinance: Insights from the 2017 Global Symposium on Microfinance (worldbank.org)
- Credit Scoring in the Era of Big Data, Yale Law School
- Use of Alternative Data to Enhance Credit Reporting to Enable Access to Digital Financial Services by Individuals and SMEs operating in the Informal Economy, INTERNATIONAL COMMITTEE ON CREDIT REPORTING (ICCR)
- Account Aggregator Frequently Asked Questions (sahamati.org.in)
About The Author
Mohit Arora is a product manager who is deeply passionate about building customer-centric products which lie at the cusp of finance & technology, harnessing data and collaborating across teams to develop propositions, strategies and processes that are highly relevant and deliver tangible value.
In his present role, Mohit is leading a product team at PayU Credit which is a credit arm of PayU in India and owns the brands of LazyPay and PaySense.